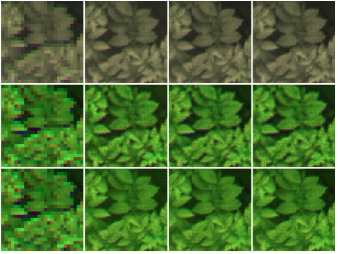
Precision agriculture using unmanned aerial vehicles (UAVs) is gaining popularity. These UAVs provide a unique aerialperspective suitable for inspecting agricultural fields. With the use of hyperspectral cameras, complex inspection tasks arebeing automated. Payload constraints of UAVs require low weight and small hyperspectral cameras; however, such cameraswith a multispectral color filter array suffer from crosstalk and a low spatial resolution. The research described in this paperaims to reduce crosstalk and to increase spatial resolution using convolutional neural networks. We propose a similaritymaximization framework which is trained to perform end-to-end demosaicking and crosstalk-correction of a 4 × 4 rawmosaic image. The proposed method produces a hyperspectral image cube with 16 times the spatial resolution of the originalcube while retaining a median structural similarity (SSIM) index of 0.85 (compared to an SSIM of 0.55 when using bilinearinterpolation). Furthermore, this paper provides insight into the beneficial effects of crosstalk for hyperspectral demosaickingand gives best practices for several architectural and hyperparameter variations as well as a theoretical reasoning behindcertain observations.
K. Dijkstra, J. van de Loosdrecht, L. R. B. Schomaker, M. A. Wiering
Published in Machine Vision and Application, 2018