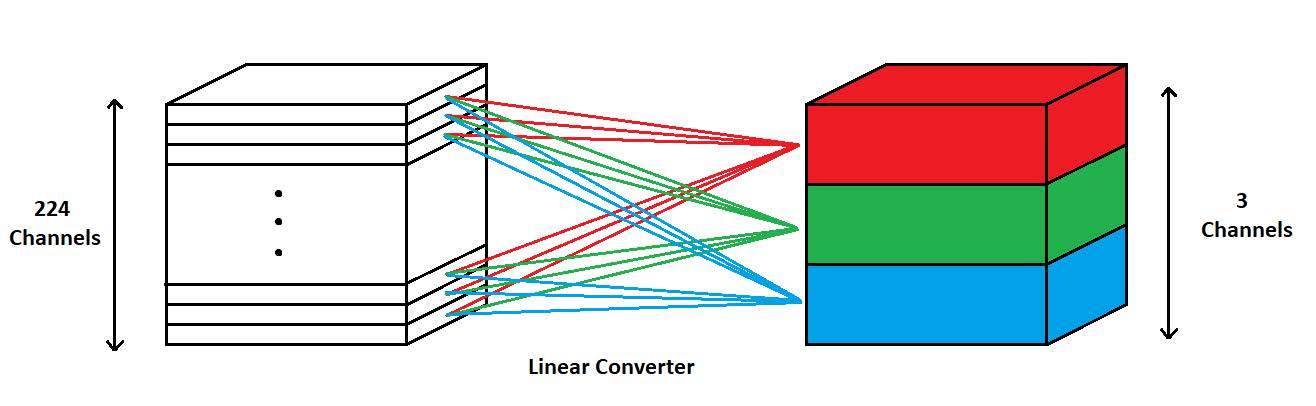
While the amount of disposed plastic waste is constantly increasing, the issue of recycling plastics largely remains an open problem. This is partially due to the complexity of distinguishing between different types of plastics. With the use of the Specim FX17 camera which operates on the near-infrared region of the light spectrum we are able to capture information unseen to the human eye, this information will be analyzed with the use of deep learning. The aim of this project is to take further steps towards the automation of plastic waste sorting. This research compares the performance of three CNNs (YOLO-V3, EfficientDet and Faster-RCNN) to see which network performs the best when it comes to the detection and classification of polymer flakes. In this research we will perform object detection on NIR hyperspectral images with the use of convolutional neural networks. These hyperspectral images contain 224 spectral layers and thus contain a lot more information to work with that is normally unseen by the human eye. The method used in this project sets up a linear converter at the network input which converts n-channeled hyperspectral images to 3-channel images thus allowing the networks to perform object detection on hyperspectral images. Experiments were conducted to see if the size of the dataset influenced the performance of the networks, if adding data augmentation improved the results and if modifying parameters such as the learning rate and the patience of the networks increased their performance. In the end we concluded that all of the aforementioned experiments did indeed give quantitative results of the best performing network. The final conclusion is that the object detection approach is worth researching further to refine the current results and that it will be a good addition to the automation of plastic waste sorting.