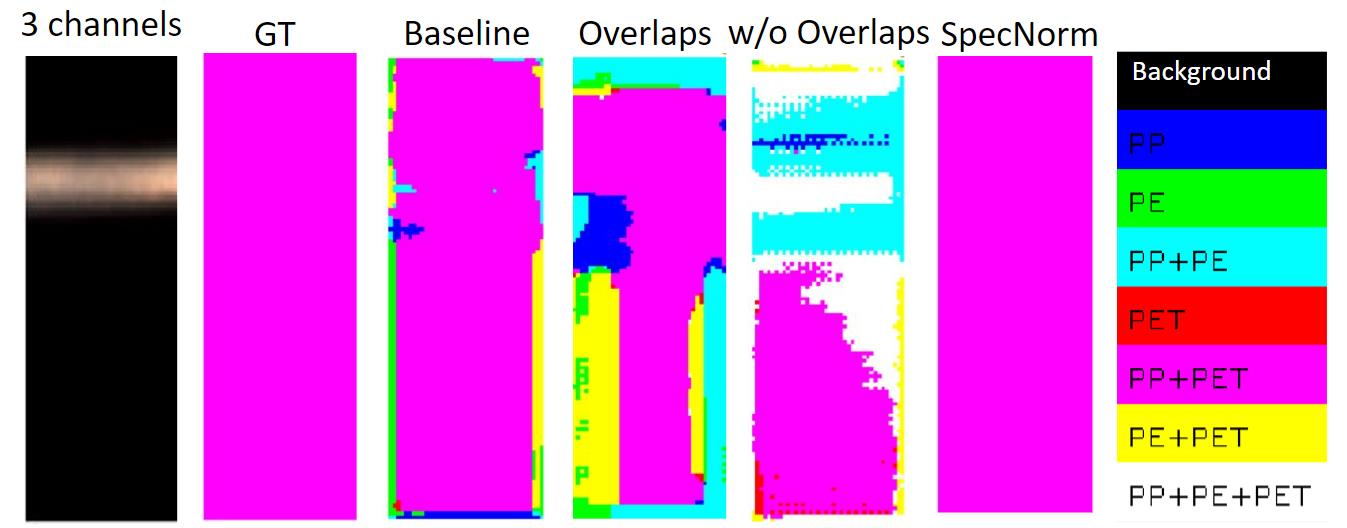
We use plastics for a large variety of tasks. However, they are highly dangerous for the environment. By recycling, we can reduce the environmental footprint of this resource. Hyperspectral Imaging is commonly used in recycling processes because some polymers are differentiable by the spectral reflectance in the Near Infra-Red range. In this research, we use hyperspectral images to segment three polymer types: PP, PE, PET and their overlapping combinations. We work with two datasets containing areas with polymer flakes overlapping. We propose bitfield encoding, an intuitive multi-label encoding approach for overlaps. With bitfield encoding, models can predict a primary classes vector and recover an overlap prediction. We use it for polymer flakes segmentation, where some polymers might overlap. For this task, we train a U-net segmentation model with bitfield encoding. It reached the same performance as a baseline approach without this encoding. We experimented with bitfield encoding by training a model with only primary classes and the task was to predict overlaps. Bitfield encoding did not achieve this goal. However, the predictions showed that, in the overlap areas, the polymers composing the overlap were predicted separately. Moreover, we used spectral normalization, a preprocessing step that removes lightning reflection or over exposition from images.
Click here for the poster of this project.