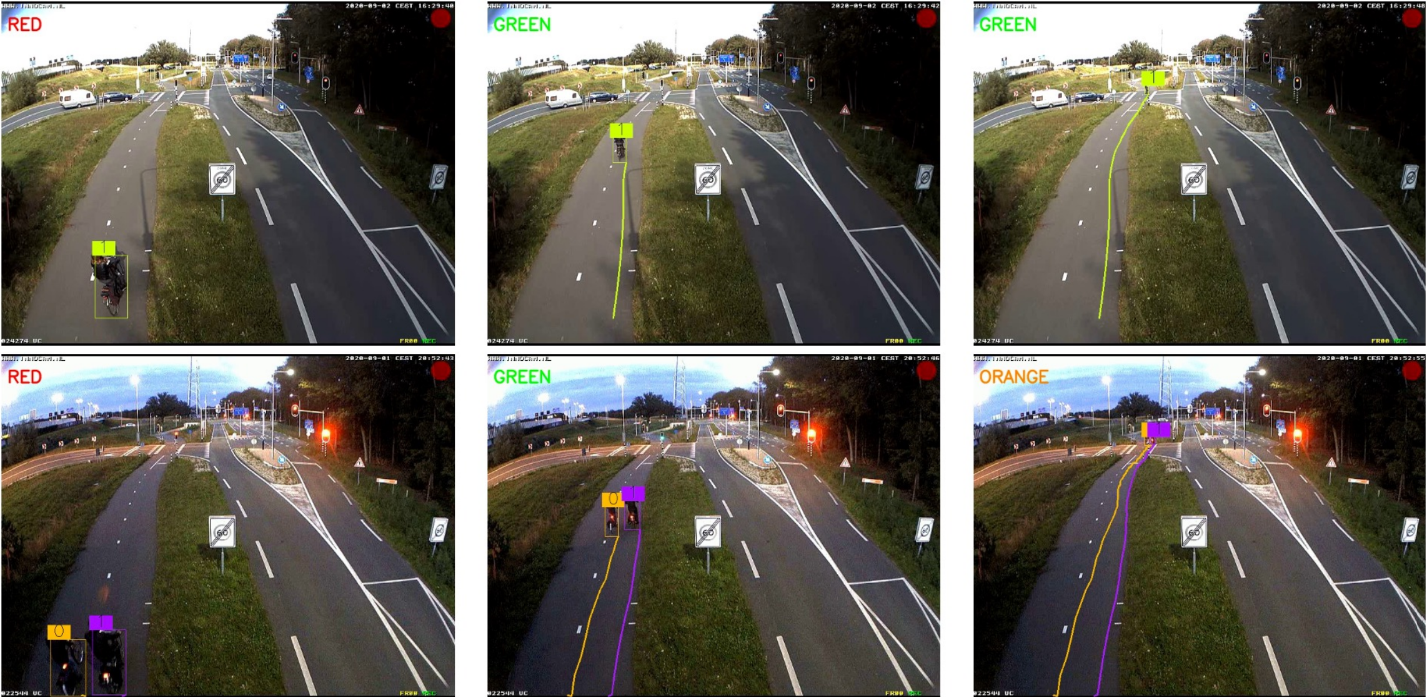
In this paper, we explore the effect of domain adaptation and catastrophic forgetting in conjunction with multiple object tracking and its application of two-wheeler tracking to see if the order of datasets during training matters. We employ a Siamese Multiple Object Tracker (SiamMOT) and train it using different permutations on two public datasets (Multiple Object Tracking (MOT) and Specialized Cyclists Dataset (SCD)) and a proprietary dataset (Traffic Intersection Dataset (TID)). We ran experiments on the datasets using different permutations to test the performance when trained on a single dataset, combining datasets and sequence training. We also qualitatively tested the generalizability of the best model in dusk/night footage. Training exclusively on the TID dataset results in the highest IDF1-score, and combining datasets results in a lower IDF1-score compared to when training exclusively on TID. Catastrophic forgetting occurs when training the model with datasets in different orders, where swapping orders of datasets leads to a reduction of about 30% in performance. We have shown that the order of datasets during training plays an important role when adapting datasets from different domains. The best model shows promising results when testing the generalizability on data from different conditions. The qualitative results of the best model on crossing a red light detection show the possibilities of using tracking-by-detection models for other traffic safety indicators.