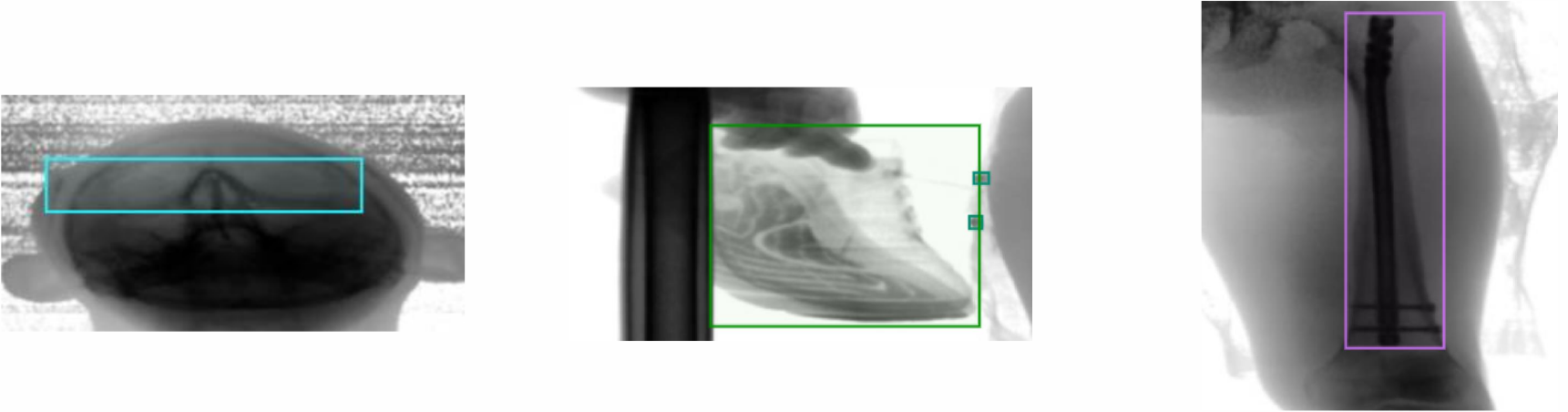
This research aims to evaluate the effectiveness of a machine learning architecture for object detection, in identifying contraband items in X-ray images. When looking for contraband, it is beneficial to identify what type of anomaly the model finds; but more importantly, where it was found. For this task, YOLOv5 was chosen as the architecture, as it is a state-of-the-art model that can locate and classify the desired objects. The model will be trained on a dataset of human X-ray images and tested on its ability to detect anomalies. The baseline model uses the full image as input and outputs predicted bounding boxes for each class. The study then conducted several experiments to address supporting questions, including the impact of data augmentation, the relationship between tile size/number of tiles and model performance, and the effect of the number of classes and class distribution on model performance. The ultimate goal is to determine the viability of using YOLOv5 to identify anomalies on x-ray images. While the results are promising, it is desirable to improve the model’s performance for the application.