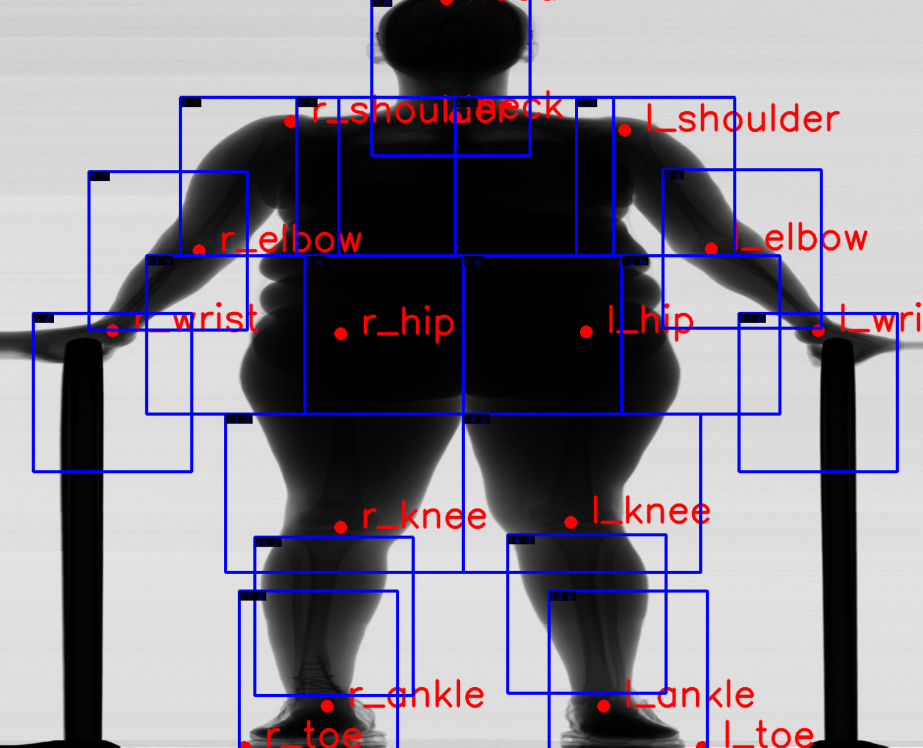
In prisons, X-ray scanners are often used to find illegal items, and anomaly detection algorithms can help human operators with additional checks. An existing unsupervised anomaly detection model uses the fixed tiling method to calculate the probability of finding an anomaly based on embedding vectors of intermediate layer outputs from the ResNet-50 backbone and multivariate Gaussian distributions. In this study, a new tiling method named “Smart Tiling” is developed to establish a relationship between each tile and a specific part of the human body in order to have homogeneous data for each tile and make finding anomalies more accurate. First, we trained a keypoint detection model to locate specific points on an X-ray image of the human body, and then tiles based on the keypoint location were generated. Experiments with different tiling and pre-processing methods are implemented, and the results of them are compared with each other. The keypoint detection model successfully worked on an X-ray image dataset to detect precise keypoints on the human body (APOKS=0.9=AROKS=0.9=0.99), and the smart tiling method produces slightly better quantitative (Recall from 0.03 to 0.04 and F1-score from 0.04 to 0.5) and qualitative results than the baseline fixed tiling method, yet it has no significant impact on the performance of the anomaly detection model.