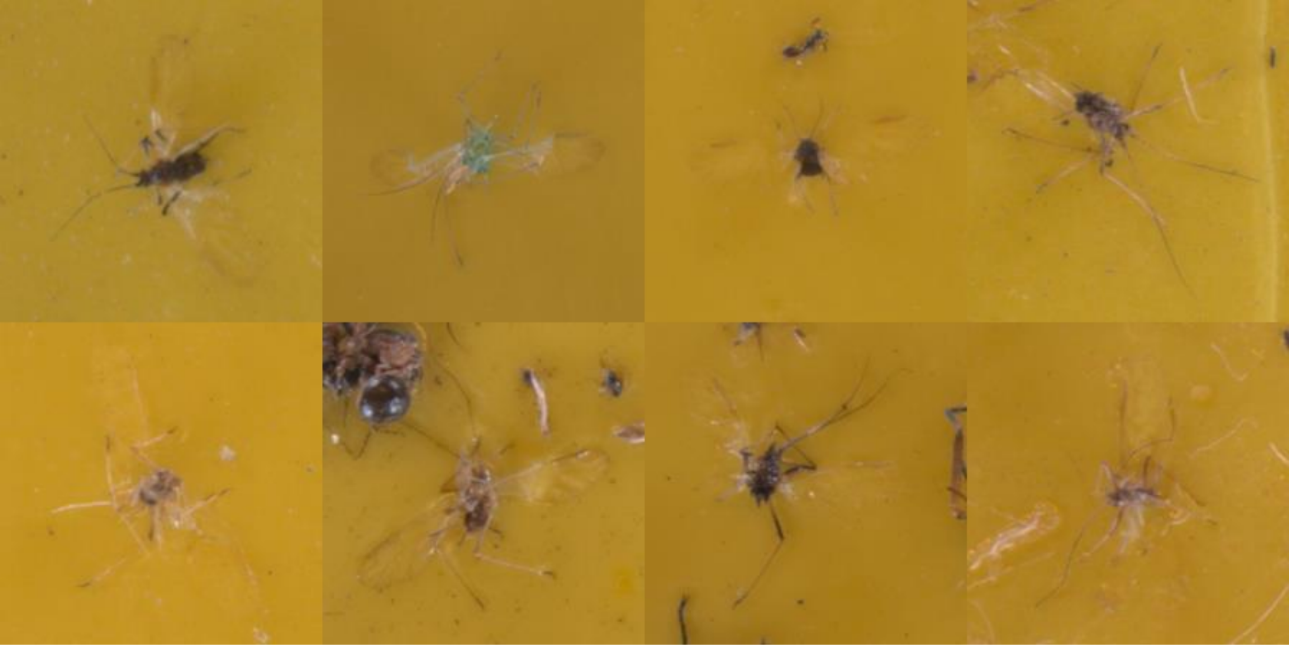
In this paper, Multi-Scale and Single-Scale architectures were evaluated to improve the classification of aphids among other insects. Early aphid identification is necessary to determine whether the presence of aphids impacts the effectiveness of pesticides. The objective is to prevent the crop (particularly seed potatoes in this study) from being contaminated with viruses transmitted by aphids. In order to classify our dataset, three distinct deep-learning models (ResNet, Vision Transformers, and Cross-Attention Multi-Scale Transformers) are evaluated. In addition to the difference in scale, two designs were included: Convolutional Neural Networks and Vision Transformers. The collected dataset used during this study contains photos of aphids and other insects. Since the difference between aphids and non-aphids is negligible, the annotations were enhanced multiple times by cleaning the data in cooperation with domain experts. Prior to the comparison, grid searches are performed on all selected models to identify the optimal parameters. The Cross-Attention Multi-Scale Vision Transformer, which is based on the Vision Transformer but expanded to a Multi-Scale architecture, achieved the greatest F1-score on aphids (84.88 percent) and lowest standard deviationamong multiple experiments (1.06 percent). The Multi-Scale method demonstrates applicable performance for classifying aphids. Several recommendations are made to further improve classification performance.