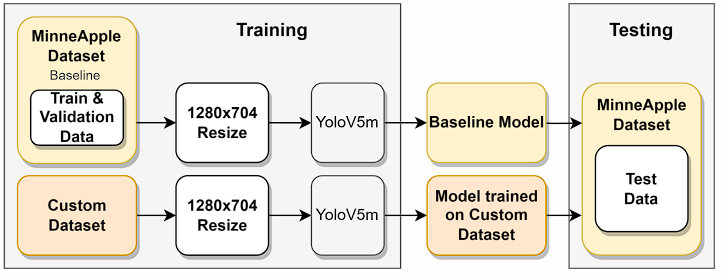
Deep object detection models have made significant strides, but the need for large training datasets remains a challenge. To address this, we explore the feasibility of using synthetic data generated by Stable Diffusion for apple tree object detection. Comparing it to a baseline model trained on real data, we create hyper realistic apple tree datasets using prompt engineering and pretrained Stable Diffusion. The annotated dataset is then used to train a YOLOv5m model [3]. Results show that the generated data model performs similarly to the baseline on real-world images, with a small average precision difference. Synthetic data generation offers potential as an alternative for training deep object detection models, reducing the reliance on extensive real data collection.